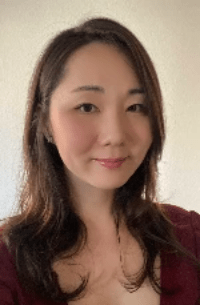
Hello!
I am a social work data scientist.
I leverage data to improve the outcomes of children and families who come to the attention of the system. My research agenda includes informing child welfare policy and practice through examination of racial and socioeconomic disparities, developing predictive models to support child welfare workers’ decision-making, and understanding the ethics and fairness of machine learning applied to child welfare.
I am an Assistant Professor at Brown School at Washington University in St. Louis. I am also an affiliated researcher at the Center for Innovation in Child Maltreatment Policy, Research and Training and Children's Data Network.
I earned my B.A. in Business from Yonsei University (Seoul, Korea), M.S.W. (Qualifying) from Monash University (Melbourne, Australia), and a doctorate degree from the Suzanne Dworak-Peck School of Social Work at the University of Southern California. I also studied at the School of Business and Management at École Supérieure de Commerce et Management (ESCEM) (Poitiers, France) as an exchange student in 2009.
Research
Leveraging data to improve the outcomes of children and families and to advance broader social goals of equity and justice
I have been dedicated to using data science to inform child welfare policy and practice ever since the field placement during my master’s program, where I witnessed how public welfare data kept in silos may hinder our efforts to serve children and families effectively. Recent advances in digital technologies and the size and richness of data in the public sector have highlighted the potential for applying data science to child welfare. Meanwhile, widely documented racial and socioeconomic disproportionality and disparity in the population of children and families who come to the attention of the child welfare system underscores the importance of the ethical use of data.
SELECTED PUBLICATIONS
- Ahn, E., Reddy, J., Rebbe, R., Palmer, L., & Putnam-Hornstein, E. (2024). Maternal Reports to the Child Protection System: A Longitudinal Analysis of Multiple Children. Child Maltreatment. https://doi.org/10.1177/10775595241281267
- Ahn, E., An, R., Jonson-Reid,M., & Palmer, L. (2024). Leveraging Machine Learning for EffectiveChild Maltreatment Prevention: A Case Study of Home Visiting Service Assessments. Child Abuse & Neglect. https://doi.org/10.1016/j.chiabu.2024.106706
- Ahn, E., Gil, Y., & Putnam-Hornstein, E. (2021). Predicting youth at high risk of aging out of foster care using machine learning methods. Child Abuse & Neglect. https://doi.org/10.1016/j.chiabu.2021.105059
- Putnam-Hornstein, E., Ahn, E., Prindle, J., Magruder, J., Webster, D., & Wildeman, C. (2021). Cumulative rates of child protection involvement and terminations of parental rights in a California birth cohort, 1999-2017. American Journal of Public Health. https://doi.org/10.2105/AJPH.2021.306214
Experiences
This interview highlights my dedication to applying data science to child welfare and my collaborative work with the First 5 Orange County Children and Families Commission.
Our Data Science for Social Good team partnered with the Memphis Fire Department (MFD) to predict individuals at high risk of becoming frequent utilizers of emergency medical services (EMS).
The Data Science for Social Good Fellowship is designed to train aspiring data scientists worldwide to work on social impact projects using machine learning and artificial intelligence in a fair and equitable manner; I was the only social work student accepted into the fellowship in the 7 years since its creation.I shared my work at Data Days Virtual 2020
My interview with the Center for Knowledge-Powered Interdisciplinary Data Science (CKIDS). An Interdisciplinary Campus-wide Research Unit
How did I become a data scientist as a non-cs major?
I have been often asked how I become a data scientist without a computer science background. Here is how.
1Explore Opportunities
Since I decided to learn data science back in 2015, I have talked to a number of experts in both social work and computer science domains to understand whether I could contribute to both fields by learning data science.
I participated in Melbourne Datathon 2016, met computational social science professors at Monash University, reached out to my current advisor Dr. Emily Putnam-Hornstein, and consulted with Social Work professors at Monash University.2Learn Programming
During my MSW program, I learned introductory data science using online MOOC courses.
Coursera
• Introduction to Probability and Data with R
• Reasoning, Data Analysis, and Writing
• Getting and Cleaning Data using R
• R Programming
• The Data Scientist's Toolbox
MIT Open Courseware
• Linear Algebra, Course # 18.06, Prof. G. Strang
iTunesU
• Statistics 110: Probability, Prof. J. Blitzstein
I also took data science electives during my doctoral training.
• Introduction to Computational Thinking and Data Science
• Principles of Programming for Informatics (See my final project here)
• Machine Learning for the Health Sciences
Of course, this was not easy, but my goal was to expose myself to data science related materials as much as possible!
3Get Trained
I learned about the Data Science for Social Good Fellowship back in 2015 when I first decided to do data science. I developed my programming knowledge for years, applied for the program in 2019, and got accepted! I was the only social work student accepted into the fellowship in the 7 years since its creation. This program provided me with great support and training.
4Apply Data Science
I have used data science in my qualifying exam and dissertation, which seeks to better support child welfare professionals with their decision-making with a focus on ML fairness.
Contact Me
Please use the following form to send a message or email me directly (ahne [at] wustl.edu). I will respond as soon as I am able. Thank you!